1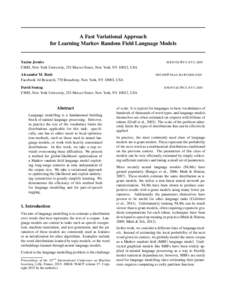 | Add to Reading ListSource URL: people.seas.harvard.eduLanguage: English - Date: 2018-07-02 10:24:03
|
---|
2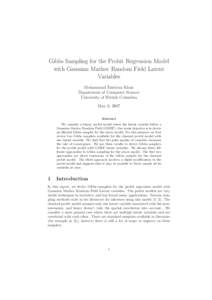 | Add to Reading ListSource URL: emtiyaz.github.ioLanguage: English - Date: 2018-08-03 01:10:16
|
---|
3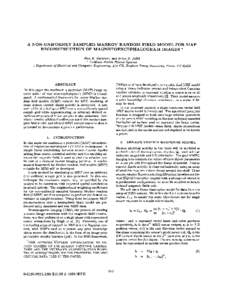 | Add to Reading ListSource URL: www.et.byu.edu- Date: 2009-10-14 12:22:26
|
---|
4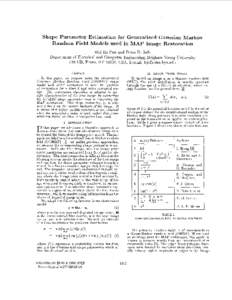 | Add to Reading ListSource URL: www.et.byu.edu- Date: 2009-10-14 12:22:27
|
---|
5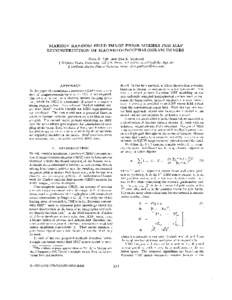 | Add to Reading ListSource URL: www.et.byu.edu- Date: 2009-10-14 12:22:28
|
---|
6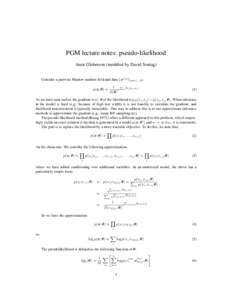 | Add to Reading ListSource URL: cs.nyu.edu- Date: 2015-11-17 16:00:53
|
---|
7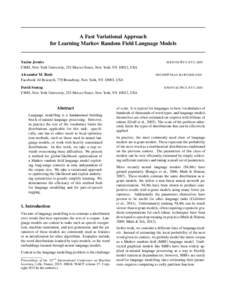 | Add to Reading ListSource URL: www.jmlr.org- Date: 2015-09-16 19:38:45
|
---|
8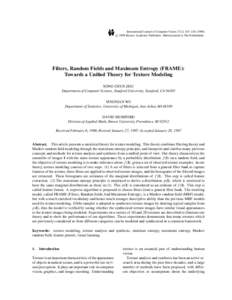 | Add to Reading ListSource URL: www.stat.ucla.eduLanguage: English - Date: 2001-02-25 14:00:10
|
---|
9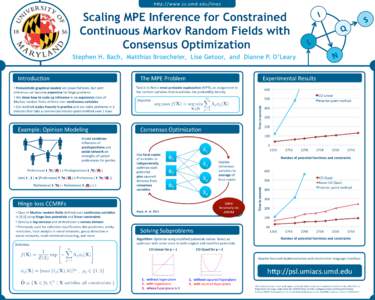 | Add to Reading ListSource URL: psl.umiacs.umd.eduLanguage: English - Date: 2013-06-14 19:26:47
|
---|
10 | Add to Reading ListSource URL: mi.eng.cam.ac.ukLanguage: English - Date: 2016-03-11 05:30:19
|
---|