1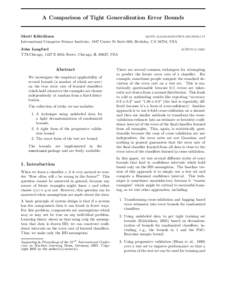 | Add to Reading ListSource URL: hunch.netLanguage: English - Date: 2005-06-01 23:11:48
|
---|
2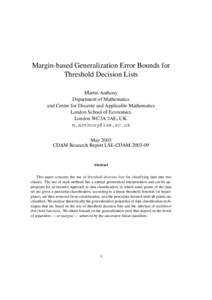 | Add to Reading ListSource URL: www.cdam.lse.ac.ukLanguage: English - Date: 2017-04-12 10:30:30
|
---|
3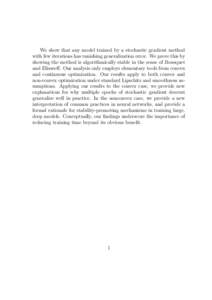 | Add to Reading ListSource URL: mmds-data.org- Date: 2016-06-23 15:50:48
|
---|
4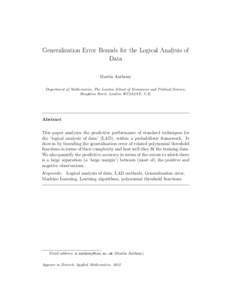 | Add to Reading ListSource URL: www.maths.lse.ac.uk- Date: 2013-01-25 06:10:29
|
---|
5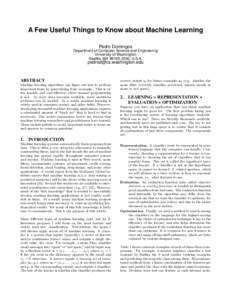 | Add to Reading ListSource URL: homes.cs.washington.eduLanguage: English - Date: 2015-08-13 22:08:12
|
---|
6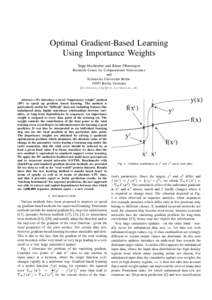 | Add to Reading ListSource URL: www.bioinf.jku.atLanguage: English - Date: 2013-01-23 02:39:45
|
---|
7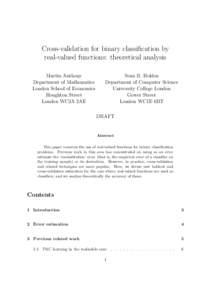 | Add to Reading ListSource URL: www.maths.lse.ac.ukLanguage: English - Date: 2000-04-03 14:24:41
|
---|
8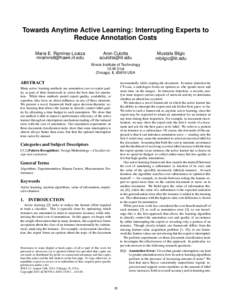 | Add to Reading ListSource URL: poloclub.gatech.eduLanguage: English - Date: 2013-07-08 06:03:55
|
---|
9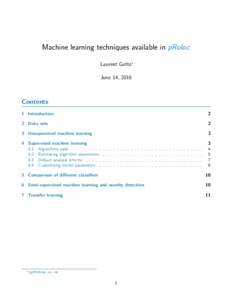 | Add to Reading ListSource URL: www.bioconductor.orgLanguage: English - Date: 2016-06-14 23:40:44
|
---|
10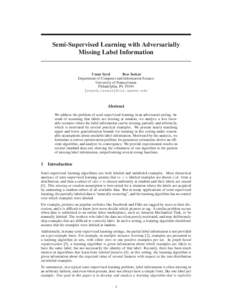 | Add to Reading ListSource URL: www.seas.upenn.eduLanguage: English - Date: 2010-11-04 10:51:45
|
---|