1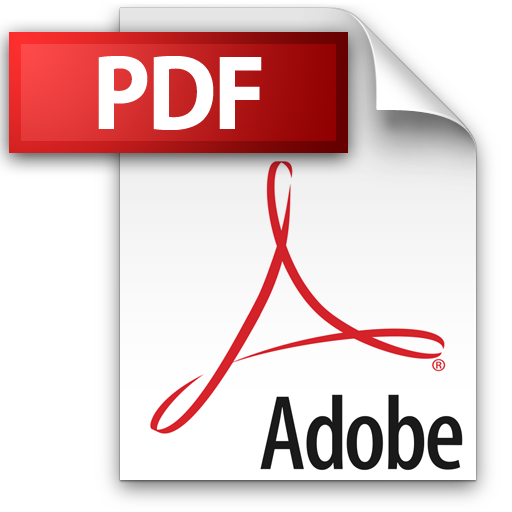 | Add to Reading ListSource URL: www.me.cs.scitec.kobe-u.ac.jpLanguage: English - Date: 2018-02-04 05:46:56
|
---|
2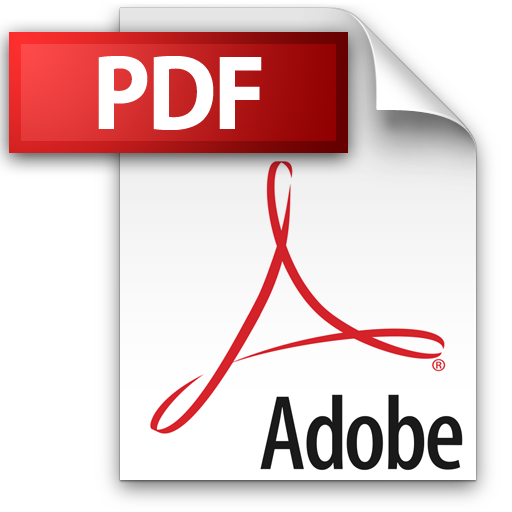 | Add to Reading ListSource URL: www.siplab.fct.ualg.ptLanguage: Portuguese - Date: 2013-12-27 03:51:55
|
---|
3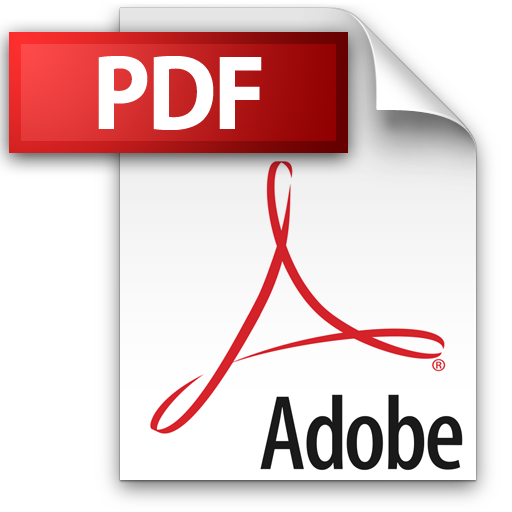 | Add to Reading ListSource URL: www.cstr.inf.ed.ac.ukLanguage: English - Date: 2015-09-29 11:06:25
|
---|
4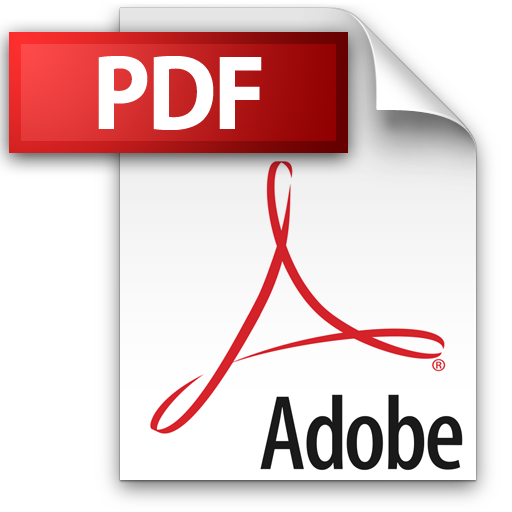 | Add to Reading ListSource URL: www.mp3-tech.orgLanguage: English - Date: 2009-06-28 10:51:53
|
---|
5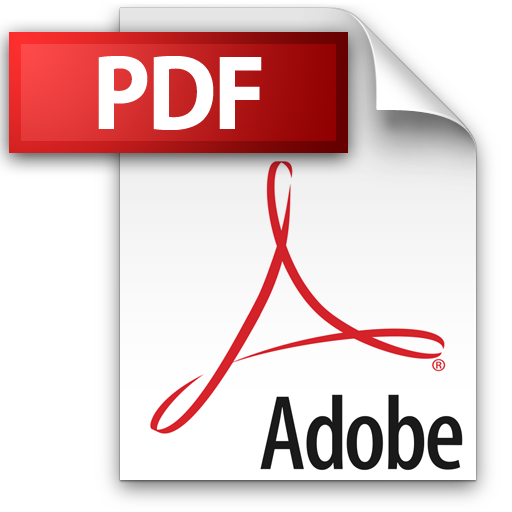 | Add to Reading ListSource URL: www.cstr.inf.ed.ac.ukLanguage: English - Date: 2015-09-29 11:06:25
|
---|
6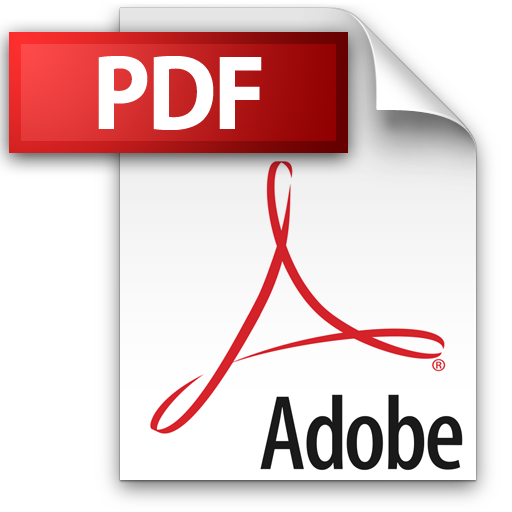 | Add to Reading ListSource URL: www.ioccp.orgLanguage: English - Date: 2016-04-05 04:56:08
|
---|
7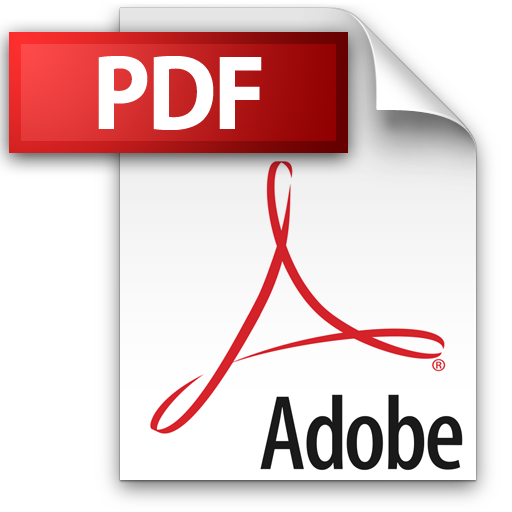 | Add to Reading ListSource URL: www.siplab.fct.ualg.ptLanguage: English - Date: 2012-12-19 07:39:10
|
---|
8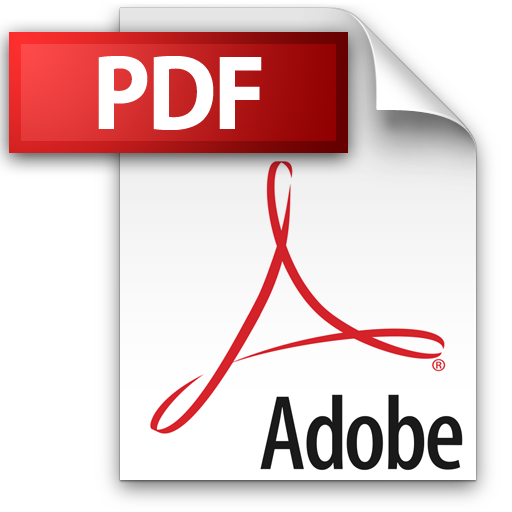 | Add to Reading ListSource URL: www.rhythmos.orgLanguage: English - Date: 2016-02-09 05:50:48
|
---|
9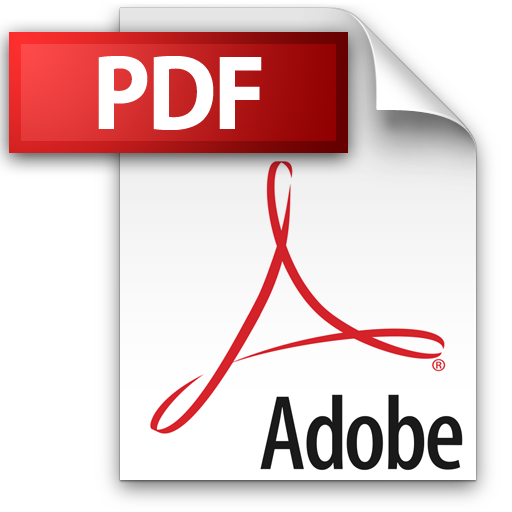 | Add to Reading ListSource URL: www.siplab.fct.ualg.ptLanguage: English - Date: 2013-10-20 12:32:13
|
---|
10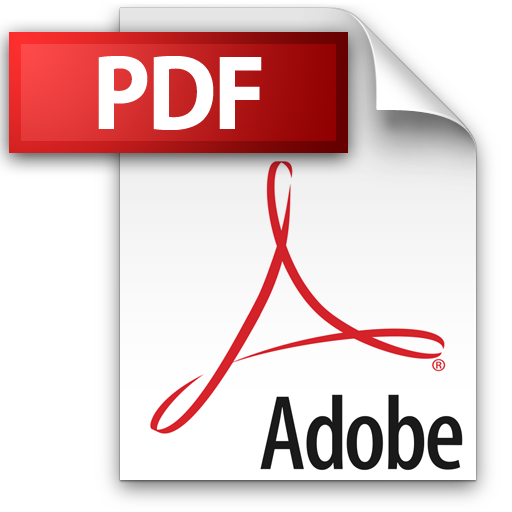 | Add to Reading ListSource URL: www.cstr.inf.ed.ac.ukLanguage: English |
---|