31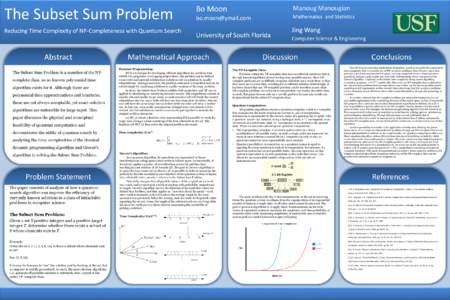 | Add to Reading ListSource URL: ciim.usf.edu- Date: 1980-01-01 00:00:00
|
---|
32 | Add to Reading ListSource URL: repository.readscheme.org- Date: 2010-10-22 08:06:07
|
---|
33 | Add to Reading ListSource URL: www.seattlescrabble.org- Date: 2015-02-16 20:46:29
|
---|
34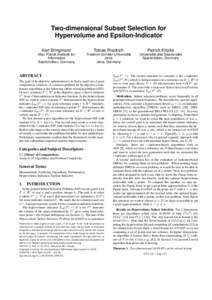 | Add to Reading ListSource URL: people.mpi-inf.mpg.de- Date: 2016-01-03 06:46:36
|
---|
35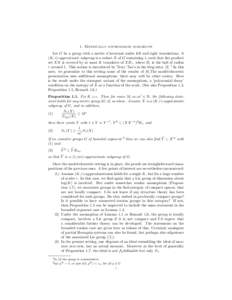 | Add to Reading ListSource URL: www.ma.huji.ac.il- Date: 2014-08-22 07:10:31
|
---|
36 | Add to Reading ListSource URL: mmds-data.org- Date: 2016-06-23 15:50:48
|
---|
37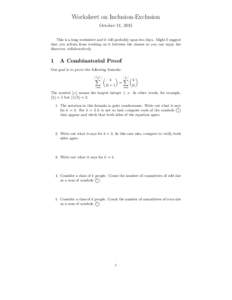 | Add to Reading ListSource URL: math.colorado.eduLanguage: English - Date: 2015-12-04 19:21:55
|
---|
38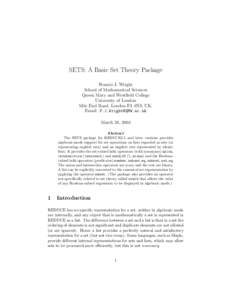 | Add to Reading ListSource URL: reduce-algebra.comLanguage: English - Date: 2008-12-30 11:47:48
|
---|
39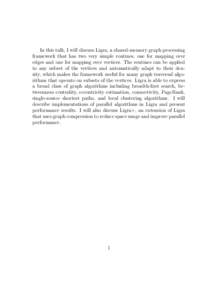 | Add to Reading ListSource URL: mmds-data.org- Date: 2016-06-23 15:50:48
|
---|
40 | Add to Reading ListSource URL: web.stanford.eduLanguage: English - Date: 2014-04-18 04:16:48
|
---|