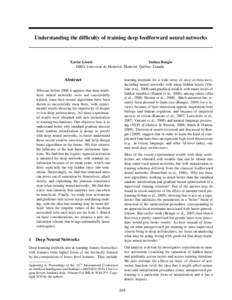
| Document Date: 2010-03-31 18:50:33 Open Document File Size: 1,57 MBShare Result on Facebook
City Courville / New York / / Company Solla S. A. / Neural Networks / MIT Press / Weston / / Country United States / Italy / / Facility Carnegie Mellon University / University of Toronto / The Robotics Institute / / IndustryTerm softsign networks / hyperbolic tangent network / hyperbolic tangent networks / investigative tool / online learning scenario / Online Learning / energy-based model / deep dense networks / deep networks / online training / natural language processing / deeper networks / softsign network / initialized network / online setting / sigmoid networks / tanh network / tanh networks / learning algorithm / deep feedforward networks / / MarketIndex set 300 / / Organization Robotics Institute / MIT / Carnegie Mellon University / University of Toronto / Pattern Analysis and Machine Intelligence / / Person Xavier Glorot Yoshua Bengio / / ProvinceOrState Sardinia / / PublishedMedium IEEE Transactions on Pattern Analysis and Machine Intelligence / Machine Learning / Complex Systems / The Journal of Machine Learning Research / / Technology natural language processing / neural network / artificial intelligence / Machine Learning / / URL http /
SocialTag |