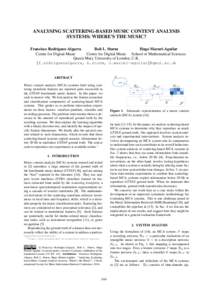 Date: 2016-08-16 16:20:31Algebra Physics Statistics Linear algebra Multivariate statistics Statistical classification Support vector machine Machine learning Euclidean vector Principal component analysis Light scattering Scattering | | – the FoM for “rock” appears to have become very poor now. • Combining all feature dimensions from acoustic information below 20 Hz and above 4186 Hz. – (Rock recovers partly) 3.3MUSIC FilteringCONTENTAdd to Reading ListSource URL: wp.nyu.eduDownload Document from Source Website File Size: 2,89 MBShare Document on Facebook
|