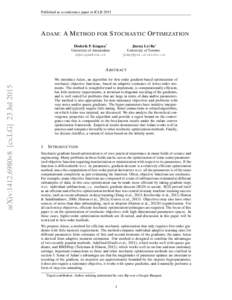 Date: 2015-07-26 20:01:45M-estimators Computational statistics Computational neuroscience Estimation theory Stochastic optimization Stochastic gradient descent Deep learning Artificial neural network Convolutional neural network Mathematical optimization Loss function Feature learning | | Published as a conference paper at ICLRA DAM : A M ETHOD FOR S TOCHASTIC O PTIMIZATION Diederik P. Kingma* University of AmsterdamAdd to Reading ListSource URL: arxiv.orgDownload Document from Source Website File Size: 605,02 KBShare Document on Facebook
|