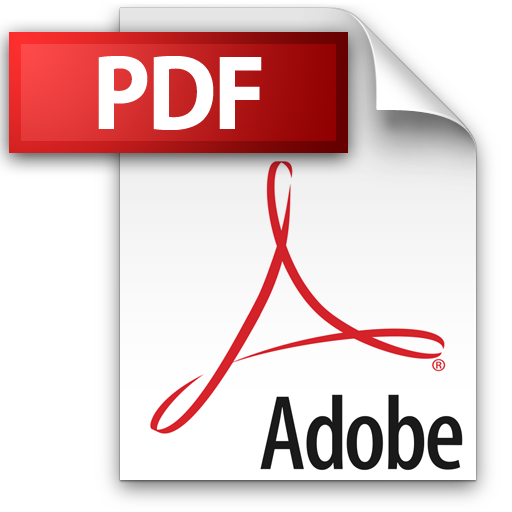 Date: 2009-08-30 09:25:51Estimation theory Computational statistics Monte Carlo methods Bayesian statistics Mixture model Markov chain Monte Carlo Markov chain Mixture distribution Gibbs sampling Statistics Probability and statistics Markov models | | Label Switch in Mixture Model and Relabeling Algorithm Project for Reading Course Prepared by: Fanfu Xie, ZhengFei Chen Label Switch in Mixture Model and Relabeling AlgorithmAdd to Reading ListSource URL: probability.caDownload Document from Source Website File Size: 1,14 MBShare Document on Facebook
|